[ad_1]
Two modes of information assortment had been designed, one for the group (i.e., the non-experts), and one for the consultants, which was based mostly on a session course of with native consultants within the nation (Fig. 1). The designs thought-about Human-Laptop Interplay trade-offs12 between annotation effectivity, annotation high quality, company of the annotators (i.e., consumer expertise when performing the duty), gamification components13, and the engineering efforts required. For the crowdsourced annotation (carried out by non-experts, or henceforth known as the group annotators), which was undertaken in a cellular utility, the duty was to just accept or reject a beforehand assigned LC label based mostly on a simplified seven class LC legend. Annotators had been requested “Do you see <LC label> in additional than half of the image?”, which they answered with “Sure”,”No”, or”Possibly”.
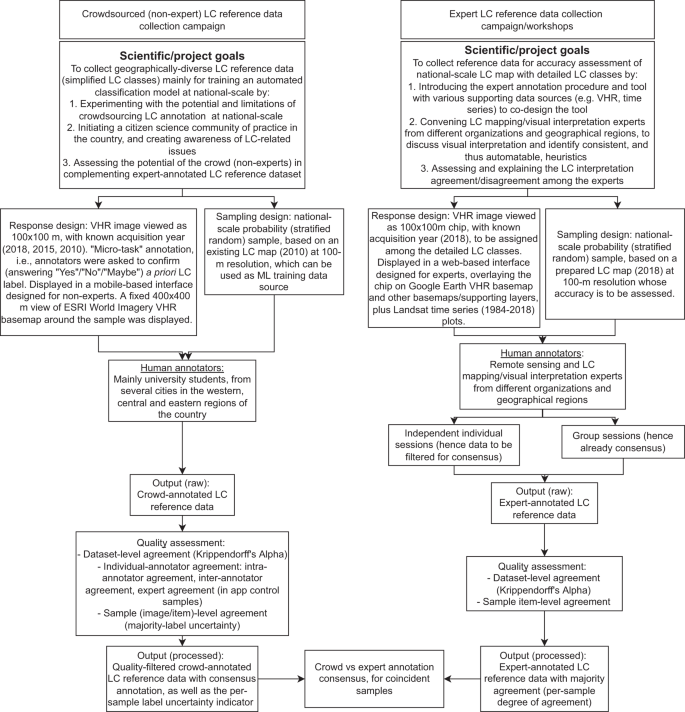
Schematic overview of the general research and information assortment design.
In distinction, the skilled annotation job (carried out by native consultants, or henceforth known as the skilled annotators) was to pick an LC label from an inventory of seventeen pre-defined LC courses from inside an internet utility. Each the crowdsourced and skilled annotations had been based mostly on interpretation of 100-by-100 m chips from very excessive spatial decision (VHR) satellite tv for pc photos offered by the Digital Globe Viewing Service, the place every chip was checked by a number of annotators to allow a consensus-based strategy2 (or “crowd fact”14) in arriving on the ultimate LC label with excessive confidence. Furthermore, a whole-systems strategy15 was adopted during which skilled workshops (for skilled annotators) and on web site outreach occasions (for crowd annotators) had been held to immediately interact with the annotators to introduce the undertaking, the scientific targets of the info assortment and the end-to-end information assortment, processing, and dissemination course of. Additional particulars of the group annotation and skilled annotation duties are offered under.
Crowdsourced LC reference information assortment by non-experts (crowd annotations)
The primary goal of the crowdsourced annotation was to generate reference information for coaching a supervised LC classification algorithm to provide a wall-to-wall map utilizing satellite tv for pc information at nationwide scale, in a separate job within the undertaking. A scientific pattern of factors spaced two km aside masking all of Indonesia was used to find out the provision of VHR imagery from the Digital Globe viewing service. From this, a stratified random pattern (proportionally allotted by class space) was derived based mostly on an current, thematically detailed LC map for 2010. The pattern allocation was moreover made proportional to the realm of seven broad geographical areas (i.e., the primary islands). The proportional allocation was based mostly on stakeholder suggestions relating to the significance of getting extra reference information in LC courses with massive areas. The whole variety of VHR picture chips acquired was based mostly on the out there funds, which coated a variety of years, i.e., 2018, for producing a more moderen LC map; 2015, which was a yr with an intense hearth season to be examined as per the suggestion of a neighborhood stakeholder; and 2010, which can be used to enhance the present reference LC map.
The LC labels offered to the non-experts had been based mostly on the reference LC map from 2010. The unique, detailed LC courses had been simplified into the next seven generic LC courses: (1) Undisturbed Forest; (2) Logged Over Forest; (3) Oil Palm Monoculture; (4) Different Tree Based mostly Methods; (5) Cropland; (6) Shrub; and (7) Grass or Savanna. This simplification was made to match the anticipated abilities of the non-expert annotators, i.e., undergraduate college students from any self-discipline at native universities within the nation. To advertise participation, ideally by these conversant in native landscapes, the native companions from the World Assets Institute (WRI) Indonesia, the World Agroforestry Centre (ICRAF) Southeast Asia Regional Workplace in Indonesia, and the World Wildlife Fund (WWF) Indonesia carried out outreach actions at sixteen Indonesian universities (10 universities in South Sumatra, 4 universities in East Kalimantan and a pair of universities in West Papua) with college students coming from many areas of the nation. Within the cellular app, the annotators may choose the placement during which the annotation duties could be positioned from a set of broader geographical areas to align with their native data if desired. As well as, they might choose the LC class to be verified i.e., the picture “pile”.
Within the cellular app (Fig. 2), the annotators had been supplied with a gallery of instance picture chips with the proper annotation and an evidence of why. To forestall poor high quality annotations or insincere/malicious participation, a quality-control mechanism was carried out within the app in the course of the crowdsourcing marketing campaign. This was carried out by randomly exhibiting management (or expertly-annotated) photos throughout every sequence of ten photos. The annotators then obtained suggestions on whether or not their annotation agreed with the skilled annotation; they had been accordingly penalised or rewarded for his or her factors as a part of the gamification technique. Efforts had been made to make sure that the management photos had been dependable and consultant of the variability of every LC sort inside every geographical area and of the solutions (“Sure”, “No”, “Possibly”). One distant sensing skilled from the nation offered the annotation on the management samples with the assistance of reference LC maps in addition to by consulting native consultants at any time when potential. In complete, the variety of distinctive management photos represented 3.06% of the overall variety of photos. Nonetheless, in follow, the variety of management photos was larger than the variety of distinctive gadgets because of two causes. Firstly, the identical management merchandise might be used as a “Sure” or “No” management merchandise for various LC courses. Secondly, management gadgets from completely different geographical areas had been used collectively to reduce the repetition of management gadgets. In follow, the management gadgets per picture pile within the cellular app had been on common 29% of all photos throughout the respective pile, with 19 out of 24 piles having greater than 10% management photos. To encourage participation, the marketing campaign offered rewards, specifically the chance to do a paid internship at both ICRAF Indonesia, WRI Indonesia, or WWF Indonesia, for the highest three annotators by way of complete rating (thus taking into consideration each amount and high quality of the annotations). A devoted web site in Indonesian was created (https://urundata.id/) to advertise the crowdsourcing marketing campaign and to assist guarantee sustainable engagement. Moreover, the outreach marketing campaign was held by means of offline seminars, webinars/workshops, and social media (i.e., Instagram @urundata and WhatsApp teams). Numerous channels had been used for the dissemination to make sure that the marketing campaign reached each goal stakeholder (i.e., college college students in metropolis areas, college college students in rural areas, researchers, and most of the people).
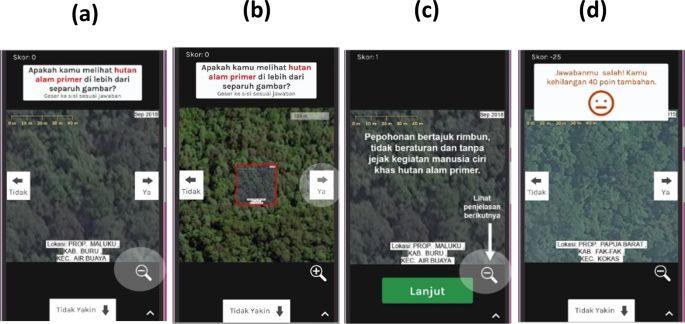
The crowdsourced non-expert LC annotation interface (in Indonesian) as a cellular utility, made out there at a devoted native web site (https://urundata.id/), which is predicated on the Image Pile critical recreation out there at https://geo-wiki.org/video games/picturepile/. (a) The annotator was proven a VHR picture chip (100-by-100 meters) with the query “Do you see <prior LC label, e.g., ‘Undisturbed Forest’> in additional than half of the image?”, which they then answered with “Sure” (swiping proper), “No” (swiping left), or “Not Certain” (“Possibly”) (swiping down). The date of the picture, the textual content stating the placement (province, district, regency) of the pattern, and a scale bar had been proven. (b) Clicking the “zoom out” icon on the backside proper in (a) opens the picture view of the larger-area (400-by-400 meters). (c) To start with the annotator went by means of instance gadgets with explanations. (d) When annotating a randomly proven management pattern (picture), the annotator obtained suggestions relating to whether or not their reply was appropriate, they usually got a bonus level or a penalty accordingly.
The crowdsourced LC annotation marketing campaign ran from 14 December 2019 to twenty-eight April 2020, throughout which a complete of two,088,515 submissions had been recorded within the cellular utility. Round 10.6% of the annotations (i.e., 221,614) had been management gadgets whereas the remaining 1,866,901 annotations had been for non-control gadgets. When aggregated to majority per annotator per merchandise, this corresponds to 928,139 distinctive annotator-unknown merchandise pairs. The marketing campaign recorded 145 days of exercise (with 136 days having greater than 100 annotations in the course of the day).
The imply variety of annotation actions per day was 14,403, with a median of 9,631 and customary deviation of 15,279, starting from 2 to 88,032 actions. The common time the annotator took to annotate an merchandise was 2.4 seconds (customary deviation 10.4 seconds, median of all actions was 1 second). A complete of 335 distinctive annotators registered and offered annotations, with the highest 10 annotators offering round 60% of all annotations, whereas the highest 5 annotators offered about 44% of all annotations (Fig. 3b). The imply variety of distinctive annotators per day was 7, with a median and customary deviation of 5 and 9, respectively, starting from 1 to 64 annotators. General, the median variety of annotations per pattern merchandise was 10.0 annotations, with a median of 11.6 and customary deviation of three.84, starting from 1 to 33 annotations (Fig. 3a). The annotations seem properly distributed throughout LC pattern pixels belonging to completely different LC courses (Fig. 3c) and geographical areas (Fig. 3d).
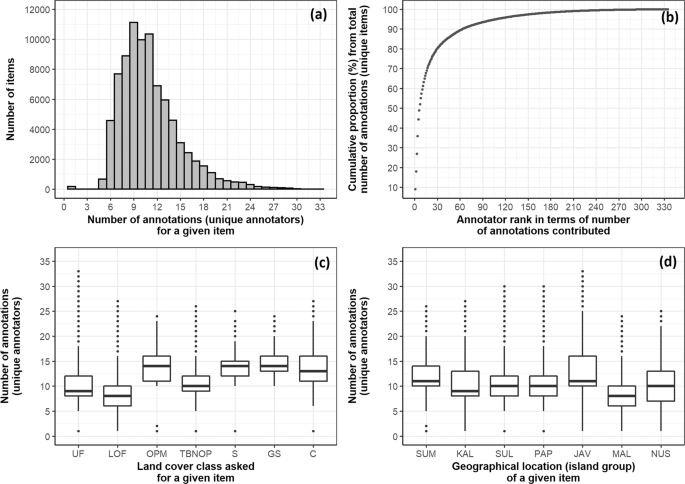
Descriptive plots describing the crowd-annotated LC reference dataset. (a) Distribution of the variety of annotations (by distinctive annotators) throughout the gadgets (samples, VHR picture chips; excluding management gadgets). (b) Distribution of the contributed annotations, for distinctive gadgets, throughout the annotators, exhibiting the contributions had been extra dominated by the highest ranked annotators. (c) Distribution of the variety of annotations (by distinctive annotators) throughout the prior LC class which the annotators had been requested to just accept/reject. UF: Undisturbed Forest; LOF: Logged Over Forest; OPM: Oil Palm Monoculture; TBNOP: Tree Based mostly Not Oil Palm; S: Shrub; GS: Grass or Savanna; C: Cropland. (d) Distribution of the variety of annotations (by distinctive annotators) throughout places (geographical areas/main island teams) of the samples. SUM: Sumatera; KAL: Kalimantan; SUL: Sulawesi; PAP: Papua; JAV: Java, Madura, Bali; MAL: Maluku; NUS: Nusa Tenggara.
LC reference information assortment by consultants (skilled annotations)
The primary goal of the skilled annotation actions was to generate reference information for performing an accuracy evaluation of a wall-to-wall LC map produced utilizing satellite tv for pc information, in a separate job within the undertaking. The LC map required an in depth legend of seventeen LC courses, and was developed for the yr 2018. From the places of obtainable VHR picture chips (see earlier part), with the acquisition yr 2018, a stratified random pattern (proportional allocation) was derived based mostly on the 2018 LC map as sampling strata. The detailed LC legend was designed along with native consultants from ICRAF Indonesia for the aim of a land restoration evaluation on the nationwide scale, taking into consideration the compatibility with current classification schemes used within the nation. Particularly, the detailed LC legend incorporates the next courses: (1) Undisturbed Dryland Forest; (2) Logged-Over Dryland Forest; (3) Undisturbed Mangrove Forest; (4) Logged-Over Mangrove Forest; (5) Undisturbed Swamp Forest; (6) Logged-Over Swamp Forest; (7) Agroforestry; (8) Plantation Forest; (9) Rubber Monoculture; (10) Oil Palm Monoculture; (11) Different Monoculture; (12) Grass or Savanna; (13) Shrub; (14) Cropland; (15) Settlement; (16) Cleared Land; and (17) Water Our bodies.
Given the complicated LC legend, the annotation job was designed for consultants. Therefore, the annotation interface offered extra help, e.g., different map layers, to find out the LC sort. A devoted department of Geo-Wiki (Fig. 4), which is a visualization, crowdsourcing and validation instrument for bettering world land cowl16,17, was developed, during which quite a few crowdsourcing campaigns have taken place prior to now4,5,6. The design of this department was knowledgeable by a workshop with native consultants. Specifically, the appliance allowed the skilled annotators to (1) freely zoom out and in (for panorama context) on the assorted VHR imagery basemaps (Google Maps Satellite tv for pc, Microsoft Bing Aerial, ESRI World Imagery); (2) view varied ancillary map layers, such because the Intact Forest Panorama layer18, a worldwide mangrove map19, an elevation layer (SRTM20), ecoregions21, the World Forest Change tree cowl loss layer22, and two layers produced by from the Joint Analysis Centre of the European Fee: World Floor Water layer23 and World Human Settlement Layer24; (3) view Landsat historic time sequence (1984–2018) of varied spectral indices; and (4) view Landsat photos for chosen dates within the companion app created utilizing the Google Earth Engine Javascript API (Fig. 5). The final function was added in consideration of the native interpreters, who’re most conversant in utilizing Landsat as major information for visible interpretation of LC, particularly within the growth of the official land cowl map by the MOEF25. The consultants had been instructed to base their LC labelling resolution totally on what’s seen within the VHR picture chip, which represents the pattern pixel and the recognized picture date.
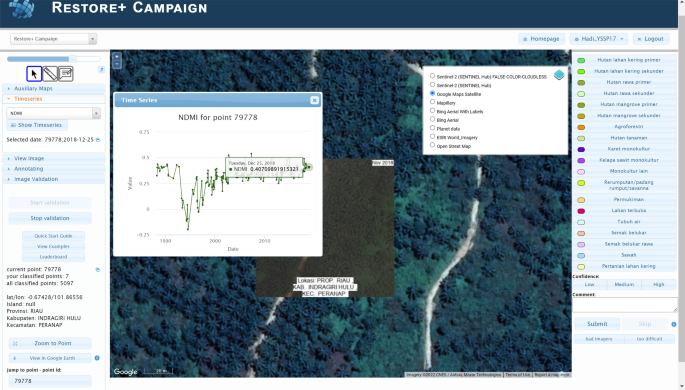
The skilled LC annotation interface (in Indonesian) as a department within the Geo-Wiki utility. The Geo-Wiki utility is on the market at https://www.geo-wiki.org/. A brief information to utilizing the appliance, which describes the primary options, is on the market at https://docs.google.com/doc/d/1CcK4BleK7N-1EnoWZKD-tHq6h49ZBQKRrO16cUIcGv0/edit. Some specific options that the consultants discovered helpful had been the power to view and freely navigate the completely different VHR picture basemaps, the Landsat time sequence and the corresponding Landsat picture, the assorted auxiliary layers akin to elevation, in addition to the power to measure distances, e.g., from roads.
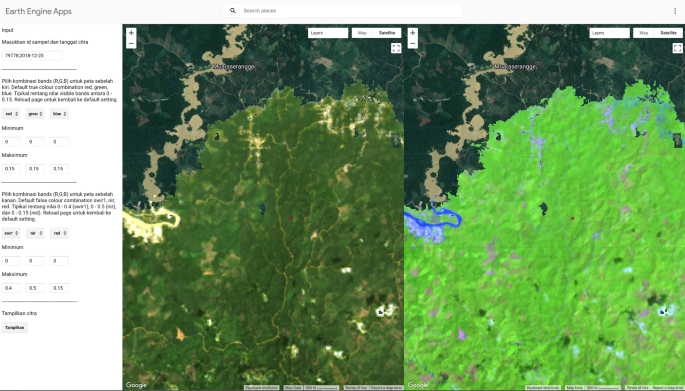
A companion Google Earth Engine (GEE) app (in Indonesian) to the skilled LC annotation course of utilizing Geo-Wiki. The GEE app permits the consultants to view Landsat photos on the location of a particular pattern for a particular Landsat remark date. The skilled annotators can customise the show of the Landsat picture. The app is on the market at https://hadicu06.customers.earthengine.app/view/restoreplus-geowiki-companion.
Direct communications with the native consultants had been established to elucidate the end-to-end research design. In the long run, the expert-annotated LC reference dataset was obtained from two sorts of interpretation session, specifically in-person workshops (known as mapathons) and particular person annotation periods. The annotations made in the course of the workshop already characterize a consensus whereas the annotations made individually by the consultants within the particular person periods wanted to be postprocessed for consensus. Within the particular person periods, to acquire LC labels with the best confidence, a minimal of three annotations had been required for every pattern. The sequence of skilled workshops introduced collectively distant sensing and LC consultants from authorities companies (i.e., Ministry of Atmosphere and Forestry; Forest Gazettement Companies from Bandar Lampung, Yogyakarta, Makassar, Palu, Banjarbaru, Manokwari, Kupang, Tanjung Pinang, and Pekanbaru; Nationwide Institute of Aeronautics and House (LAPAN); and Geospatial Data Company (BIG); Company for the Evaluation and Utility of Expertise (BPPT)), civil society organizations (i.e., Burung Indonesia; FAO; TNC; Auriga; USAID IUWASH PLUS; Forest Carbon; and Wetlands Worldwide Indonesia), and universities (College of Indonesia; Universitas Indo World Mandiri Palembang; Bogor Agricultural College; Mulawarman College; and Politeknik Pertanian Negeri Samarinda). The native consultants had been from the western, center, and jap areas of the nation (with every of the main island areas of Sumatera, Java, Kalimantan, Sulawesi, and Papua represented). Throughout the workshops, the skilled annotators had been divided into teams (Sumatera, Java-Madura-Bali, Kalimantan, Sulawesi-Maluku, and Papua-Nusa) to interpret the samples positioned in these geographical areas with which that they had most familiarity. The workshops began with a dialogue to construct a typical understanding, and thus constant, clear, goal, and reproducible interpretations2 of the LC legend and definitions, by going by means of chosen examples collectively, together with troublesome edge instances (i.e., instances falling between two land cowl courses). Throughout the workshops, energetic dialogue among the many consultants was inspired to make express their interpretation course of (notion and cognition, assumptions, visible cues, ground-based data, and so on.)26. As an incentive for energetic participation by the consultants, along with sharing the collectively produced LC reference dataset, a coaching session on LC mapping utilizing cloud computing in Google Earth Engine was offered in the course of the workshop (recordings out there in Indonesian at https://www.youtube.com/channel/UCY7fr6OtwumeIXDlWW9wd6A/movies). For the person periods, these consultants who had been capable of annotate 500 samples had been invited as co-authors on this publication.
The skilled workshops had been held on 12–13 February 2020 in Jakarta, Indonesia, adopted by an internet workshop on 10 June 2020. Within the February workshop, nineteen native and regional LC consultants had been divided into seven interpreter teams based mostly on familiarity with the geographical area. Every group of interpreters annotated between 15 and 77 samples (with a median amongst teams of 42). Every group of interpreters was accompanied by facilitators from ICRAF Indonesia. Within the on-line workshop in June, 62 native and regional LC consultants participated. Throughout the LC annotation session, 5 interpreter teams had been shaped, with every group annotating between 30 and 82 samples (with a median of 46 throughout teams). The median size of time to annotate one merchandise was 121 seconds within the February workshop, and 43 seconds within the on-line workshop in June.
The impartial annotation actions had been held between 10 June 2020 and 20 July 2020. Eleven LC consultants actively participated, and by the top of the actions, eight consultants had annotated round 500 samples every (who had been then invited as co-authors on this paper) inside 6.5 days (starting from 3 to 9 days). The median time that the collaborating consultants took to annotate one merchandise was 41 seconds, which has similarities to the group session within the on-line workshop. Within the particular person periods, 1,450 samples had three annotations, 91 samples had two annotations, and 63 samples had one annotation.
From the skilled LC reference information annotation actions, a complete of 5,187 annotations was collected. Of those, 536 annotations had been collected throughout skilled workshops/mapathons and therefore all 536 samples had been already annotated with a consensus LC label, and 4,651 annotations masking 1,618 pattern gadgets had been collected independently carried out utilizing the net utility.
Publish-hoc high quality evaluation measures for the human-annotated datasets
A recognized difficulty with crowdsourced information is the variable, and sometimes unknown, high quality. Along with the measures taken to forestall poor high quality annotation in the course of the annotation actions (akin to offering pointers, and utilizing management samples for the group annotation), we carried out strategies for post-hoc detection of poor high quality annotations27 in addition to a high quality evaluation of the annotation information on the stage of the whole dataset, particular person annotators, and particular person pattern gadgets. Using a number of high quality management measures, i.e., stability, reproducibility, and accuracy28, offers quantitative proof for the reliability of the datasets. We be aware that information high quality points in crowdsourced information, and the selection of high quality evaluation metrics, are nonetheless an open space of analysis29,30. That is additionally true within the broader AI area, i.e., at the moment there aren’t any standardized metrics for characterising the goodness-of-data31. Analysis within the Volunteered Geographic Data (VGI) area recommends the combination of a number of high quality measures to provide extra dependable high quality info1. In our analysis, we adopted the established practices on human annotation information high quality evaluation associated to inter-rater reliability (inter-rater settlement) from content material evaluation and the associated social science literature12,28. It is a follow that we encourage for additional adoption by the LC mapping neighborhood as it may be anticipated that human-annotated labels might be more and more collected to deal with coaching information bottlenecks in realizing the total potential of recent ML and AI algorithms. The basic property of high quality evaluation metrics is that they appropriate the noticed settlement for anticipated probability agreements.
We first took the bulk annotation made by an annotator for a picture that they annotated greater than as soon as. If there was no majority, we stored the final annotation that the consumer made for that picture. Dataset-level settlement was measured with the statistic known as Krippendorff’s Alpha28,32. Krippendorff’s Alpha is a generalization of a number of recognized reliability indices, which has the advantages of being relevant to any variety of annotators (not simply two), any variety of classes, and huge and small pattern sizes alike, in addition to coping with bias in disagreement, and is invariant to the selective participation of the annotators, i.e., it could possibly take care of the truth that not each merchandise is annotated by each annotator. The anticipated settlement is the info frequency. A Krippendorff’s Alpha worth of 1.00 signifies excellent reliability whereas 0.00 signifies absence of reliability28.
Particular person-annotator settlement was measured by way of intra-annotator settlement (or stability), inter-annotator settlement (or majority settlement and reproducibility), and expert-agreement (or accuracy, estimated with management samples). These metrics had been summarized by picture pile to account for the potential variability within the job problem and the annotator’s ability with respect to the LC class or/and geographical area of the picture. Intra-annotator settlement was calculated because the proportion of occasions the annotator’s annotation agreed with their earlier annotation for that very same picture. The intra-annotator settlement values per annotator and per picture had been then averaged into per annotator and per pile. Professional settlement was calculated because the proportion of occasions the annotator accurately annotated the management samples. Professional settlement was calculated by picture pile. The anticipated settlement was the info frequency12, i.e., the label frequency of the management gadgets that appeared in the course of the marketing campaign. Inter-annotator settlement was calculated because the proportion of photos on which the annotator agreed with the vast majority of annotations made by the opposite annotators for that very same picture. The anticipated settlement was probably the most frequent label of the management photos that appeared in every pile in the course of the marketing campaign. The inter-annotator settlement values per annotator and per picture had been then averaged into per annotator and per pile. The three individual-annotator settlement metrics had been thought-about collectively to evaluate the credibility of the annotator. The metrics had been calculated per picture pile within the crowd-annotated information, and thus, we account for potential variations in crowd abilities for various LC courses or/and geographical areas.
Figuring out the ultimate most assured LC annotated label for every pattern merchandise
For the crowd-annotated dataset, to find out the ultimate consensus annotation for every pattern, we aggregated the annotations based mostly on a weighted majority scheme with the credibility rating of every annotator as weights. That’s, the general confidence of every potential annotation (“Sure”, “No”, or “Possibly”) for every pattern was estimated because the sum of credibility scores (weights) of the annotators who offered every annotation respectively, divided by the sum of credibility scores of all annotators that annotated that pattern. The annotation that had the best worth of general confidence was decided as the ultimate consensus annotation, with the uncertainty of that ultimate consensus label estimated as one minus the general confidence worth (“least confidence” uncertainty sampling12). In our experiments, inferring the person annotator’s credibility based mostly on individual-annotator skilled settlement alone, and excluding annotations from annotators with a unfavourable credibility rating, was discovered to offer the best dataset accuracy as assessed with one of the best out there gold-standard reference on this research, specifically the expert-annotated information with the bulk label. For the expert-annotated dataset, the ultimate label for samples with a majority was used, i.e., for samples obtained from the group periods and from impartial particular person periods, samples with a % majority of greater than 50%.
[ad_2]
Source link