[ad_1]
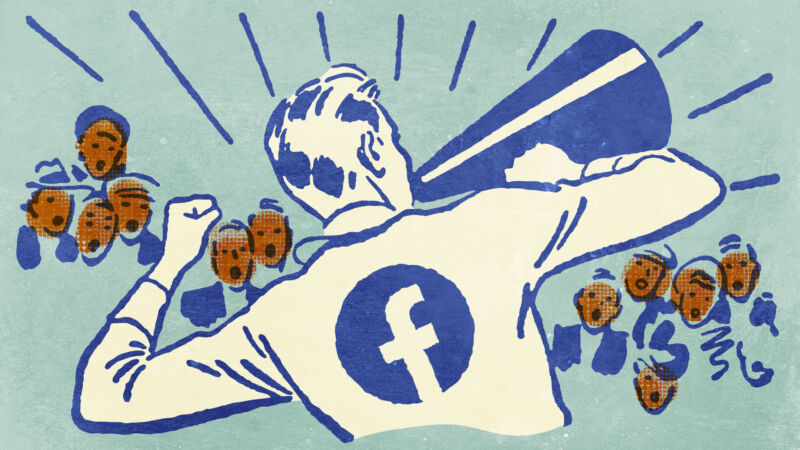
Getty Photos | Aurich Lawson
During the last a number of years, there have been rising considerations in regards to the affect of social media on fostering political polarization within the US, with important implications for democracy. Nevertheless it’s unclear whether or not our on-line “echo chambers” are the driving issue behind that polarization or whether or not social media merely displays (and arguably amplifies) divisions that exist already. A number of intervention methods have been proposed to scale back polarization and the unfold of misinformation on social media, however it’s equally unclear how efficient they might be at addressing the issue.
The US 2020 Fb and Instagram Election Research is a joint collaboration between a gaggle of unbiased exterior lecturers from a number of establishments and Meta, the mother or father firm of Fb and Instagram. The challenge is designed to discover these and different related questions in regards to the function of social media in democracy inside the context of the 2020 US election. It is also a primary when it comes to the diploma of transparency and independence that Meta has granted to tutorial researchers. Now we now have the primary outcomes from this uncommon collaboration, detailed in 4 separate papers—the primary spherical of over a dozen research stemming from the challenge.
Three of the papers had been printed in a particular subject of the journal Science. The primary paper investigated how publicity to political information content material on Fb was segregated ideologically. The second paper delved into the results of a reverse chronological feed versus an algorithmic one. The third paper examined the results of publicity to reshared content material on Fb. And the fourth paper, printed in Nature, explored the extent to which social media “echo chambers” contribute to elevated polarization and hostility.
“We discover that algorithms are extraordinarily influential in folks’s on-platform experiences, and there may be important ideological segregation in political information publicity,” Natalie Jomini Stroud of the College of Texas at Austin—co-academic analysis lead for the challenge, together with New York College’s Joshua Tucker—mentioned throughout a press briefing. “We additionally discover that well-liked proposals to alter social media algorithms didn’t sway political attitudes.”
Ideological segregation
Let’s begin with the query of whether or not or not Fb allows extra ideological segregation in customers’ consumption of political information. Sandra Gonzalez-Bailon of the College of Pennsylvania and her co-authors appeared on the conduct of 208 million Fb customers between September 2020 and February 2021. For privateness causes, they didn’t have a look at individual-level information, per Gonzalez-Bailon, focusing solely on aggregated measures of viewers conduct and viewers composition. So the URLs they analyzed had been posted by customers greater than 100 occasions.
The outcomes: Conservatives and liberals do certainly see and have interaction with completely different units of political information—sturdy ideological separation. That segregation is much more pronounced when political information is posted by pages or teams versus people. “In different phrases, pages and teams contribute way more to segregation than customers,” mentioned Gonzalez-Bailon. Moreover, politically conservative customers are way more segregated and are uncovered to way more info on Fb than liberal customers; there have been much more political information URLs seen completely by conservatives in comparison with these seen completely by liberals.
Lastly, the overwhelming majority of political information that Meta’s third-party fact-checker program rated as false was seen by conservatives, in comparison with liberals. That mentioned, these false scores amounted to a mere 0.2 p.c, on common, of the complete quantity of content material on Fb. And political information usually accounts for simply 3 p.c of all posts shared on Fb, so it is not even remotely the most well-liked sort of content material. “This segregation is the results of a fancy interplay between algorithmic types of curation and social types of curation, and these suggestions loops are very tough to disentangle with observational information,” mentioned Gonzalez-Bailon of the examine’s findings.
[ad_2]
Source link